Interview with Salma Mesmoudi on AI for the Brain and Memory
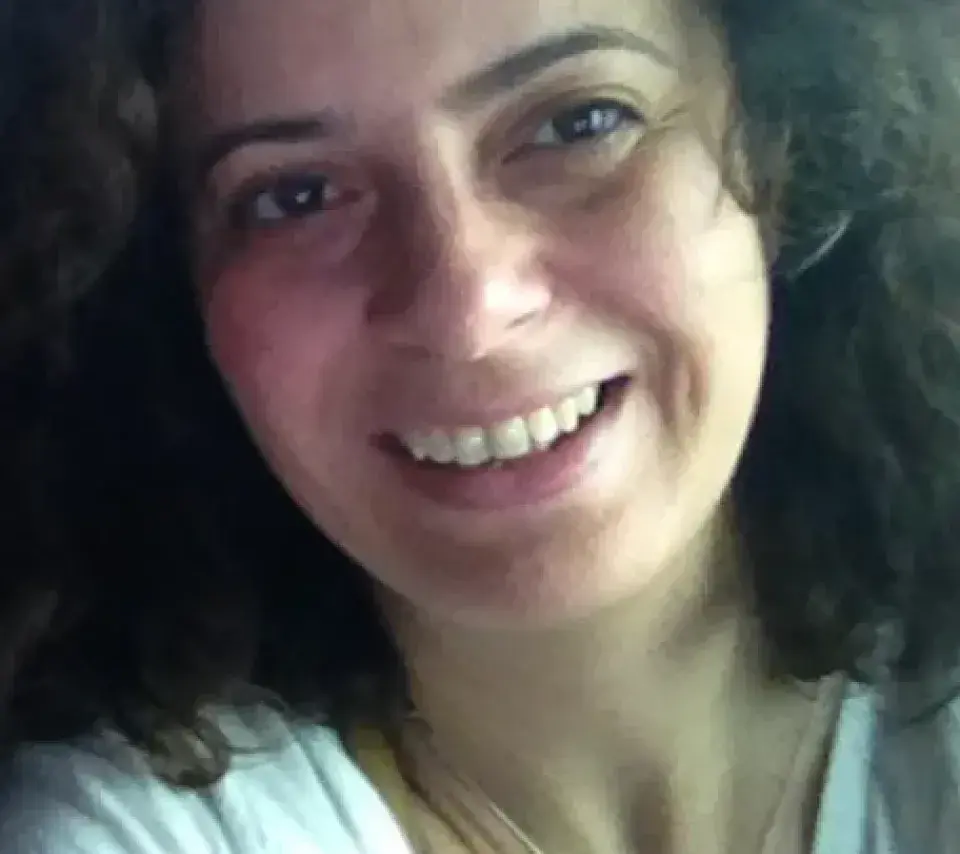
Salma Mesmoudi is an engineering researcher with the Paris 1 Panthéon-Sorbonne University. Her current research focuses on the large-scale integration of multiple-source data about the brain. This work involves the multimodal processing of magnetic resonance imaging (MRI) images, and the integration of these images both with experimental data in genetics and with large databases of bibliographical metadata. This integration of brain data has already resulted in the development of a new functional model of information processing in the brain — the dual intertwined rings model —, as well as in an online platform “https://linkrdata.fr” allowing researchers and clinicians to easily match different types of images, and to extract knowledge from their data.
She kindly discussed her professional background and her research on artificial intelligence in other fields with the Paris 1 Panthéon-Sorbonne University Artificial Intelligence Observatory.
Unprecedented changes in artificial intelligence research
Salma Mesmoudi: I obtained my PhD in artificial intelligence in 2005. At the time, artificial intelligence mostly referred to expert systems. We were still excited about the machine beating the world chess champion. There were also two other areas in the field. One of them was based on neural networks, which were mostly used in voice recognition and for other projects like computer-assisted vision. The other one, ie. evolutionary algorithms, which draw on evolutionary theory to solve various problems, has now become a dead area of study in artificial intelligence. Several of these projects were very promising — but computing power was not sufficient to launch any of them. The year 2010 was a crucial year for the field of artificial intelligence as a whole, and for my career as well. It is the year where exceptional technological progress took place, dramatically increasing computing power: we started using graphics card processors, and it simply boosted data storage and data processing. In learning-related fields, deep learning started its considerable advances, because computing speed had been multiplied by 2,000.
It was in this context that I worked at the Pitié-Salpêtrière Hospital neuroimaging laboratory as a postdoctoral student. It also coincided with the start of data sharing in neuroimaging, an initiative launched by the National Institute for Health (NIH) in the US through platforms on which brain MRI data could be collated and shared world-wide. The data can be accessed for free by academics thanks to open data sharing policies, so that researchers can benefit from all neuroimaging results generated in the world. During my postdoc at the Pitié-Salpêtrière, we wanted to take advantage of this novel data sharing to transition from our local databases — which contained at best about 40 brain MRIs — to a larger sample size of 400 brain MRIs. The task at hand required a change in scale allowing us to process 400 images at the same time. It was the first time I was working on the brain. I discovered this endless world of data, which never stops growing, where there is always new data. The first work I did involved algorithmic improvements targetting processing for 400 images. Later on, I did research to develop a proof for a new functional model for the brain. When I found out about another type of open data available to researchers — genetic data — we confirmed that our newly-published functional model also applied to a genetic scale. Bibliography databases were also turning to open data. So I began to think about the value of this data, and about how I could integrate it and allow my colleagues in the neuroimaging lab to benefit from it. Afterwards, I became interested in understanding recent algorithmic developments like natural language processing. The first step in the resulting research project concerned the brain, and was called LinkRbrain. My goal was to put together data about the complex topic of brain function coming from a variety of sources. Specific artificial intelligence algorithms were thus required to process all this data.
Using AI to expand our knowledge of the brain
Salma Mesmoudi: Later on, LinkRbrain became an open access online platform dedicated to the multi-scale integration, visualization and documentation of data on the human brain. It compiles and brings together anatomical, functional and genetic data produced by the scientific community.
When I began working in this area, we had around 40 MRIs at our disposal, and we could detect twelve different signals coming from parts of the brain. Thanks to data sharing platforms, I looked through other MRIs, and I collated the data which was statistically homogeneous. I managed to process 400 MRIs at the same time, and I found out that what had been taken for noise before was a signal. In fact, working with only 40 MRIs, we did not have enough statistical reach for this apparent noise to come through as signals. Think of a microscope: the more you increase the resolution, the more new structures we can see. The same is true for data: the greater the amount of data you work with, the more statistically relevant the results you obtain. So we went from twelve signals to 32, but we did not know what they meant. So we worked with a Pitié-Salpêtrière neuroanatomist, who dedicated entire days to our project. It was not an easy task, however, to determine precisely which brain function could be associated with which signal. We had the idea of looking at bibliographical references from the literature — but it is impossible to synthesize this amount of data by hand. For instance, only on Alzheimer’s disease, there are more than one million published entries. So we tried to extract knowledge from the data: we started with 5,000 papers, and thanks to a Prématuration grant from the Centre national de la recherche scientifique’s Innovation branch (CNRS Innovation), we were able to increase that number to 14,000. We used natural language processing algorithms (NLP) and did two rounds of exploration: regular text exploration and deeper exploration for the papers including brain coordinates. I must stress that the first brain researchers were visionaries who created an average map of the brain relying on sets of three coordinates (x, y and z). This is what enables us today, for instance, to know the coordinates of the parts of the brain used to perform mental arithmetic. All we have to do is to analyze MRI results from individuals who were asked to perform mental mathematical operations, and then to identify the more active brain regions, ie. parts which send a stronger signal than the rest. Through these spikes in brain activity, we can identify 3D coordinates for the regions involved. This means that, if we read a paper detailing the results of this experiment, we will find the name of the cognitive task which was tested for (in this case: mental calculation), and a table with coordinates corresponding to activity spikes. We repeated this process for other cognitive functions, which allowed us to synthesize the coordinates and their corresponding cognitive and sensorimotor functions. With this synthetic view, we were able to develop a refined functional map of the brain. The map tells us which coordinates are associated with which functions. In the brain, it is not one function per region. In fact, one function can activate several regions of the brain, and conversely, one region can be activated by several sensorimotor and cognitive functions. This bibliographical synthesis leads to the cognitive branch of our project.
Concerning our project’s other branch — the genetic or transcriptomical aspect —, I must mention the work done by the Allen Institute for brain science. They did magnificient work to detect gene transcription rates in the brain, identifying these rates for about 21,000 genes in roughly 1,000 brain regions in six individual brains. Just think of all the studies you could run with this data! On my part, I found their work inspiring and decided to integrate both cognitive and genetic scales to our project. I other words, I wanted to find a way to connect cognitive functions and gene transcription.
To complete this project, we designed a compendium of brain pathologies based on the literature, and another compendium for brain fibers based on a shared database of anatomical imaging and diffusion. Concerning brain fibers, let me point out that neurons are made of a neuron body and synapses. A fiber connects these two parts and transmits information — we call these fibers “information routes”. Information originates in the neuron body, and makes its way to the synapses to reach other neurons. Our idea was to map the routes, roads and highways in the brain. For instance, what are the regions where many fibers are present? Where are these fibers most dense? In other words, where are the highways? And the small country roads? Since I am not a neuroscientist but a specialist of artificial intelligence and statistics, my idea (which can seem simple at first sight) was to project cognitive functions and genomic transcriptions on a map as coordinates. So I drew on my AI skills to associate brain functions with genes, to know how and where the latter are activated or expressed. In other words, the idea was to start from a gene or a group of genes, and then observe which functions activate the regions where these genes are most expressed. And so I designed a unifying matrix in which we can go from one scale to another, thus integrating new information on the same map I had started with.
Improving the LinkRbrain project with the Matrice technological platform
Salma Mesmoudi: By 2012, we were ready to expand the project and to open it up for other researchers. Around that time, I met the members of Matrice, a technological plateform designed to study individual memory and its role in the construction of collective memory. Matrice was conceived as an interdisciplinary endeavor, ie. it studies memory from the various perspectives of history, biology, sociology, etc. They found the LinkRbrain project very interesting as a tool, since the software enables sociologists or historians who work on aspects of memory to understand memory from a biological standpoint without being trained brain specialists.
Thanks to funding from Matrice, the LinkRbrain platform now allows researchers:
- To run automated meta-analyses on all relevant papers (5,000 in total) based on selected functions, and to extract all activation tables (in Talairash and MNI coordinates) from them automatically;
- To visualize the automated meta-analysis results as comparative activation maps showing the activated brain networks for various functions involved in memory and cognition (the 3D brain map can be pivoted, zoomed into, etc.), and to better visualize all reconstructed activations (2D interactive activation maps of specialized networks are available for more accurate contrasts);
- To generate graphs for the interaction between the selected functions and all other, closest brain functions (such graphs are particularly interesting for collaborators from other fields, since they do not require prior knowledge of brain anatomy);
- To generate lists of the quantified values of all interactions between reference functions (in this case, autobiographical memory) and all other, closest brain functions;
- To make all these results available to other researchers; and
- To integrate libraries of results from external databases (especially open access databases) or from the researcher’s own experiments.
The software platform has since evolved: it now includes four interactive modules which operate from a collaborative platform for the automated integration of results in brain research, LinkRdata. These results are otherwise currently scattered among thousands of research papers and several experiment databases.
Expansion of the project to other fields: the Second World War and the November 2015 Paris attacks
Salma Mesmoudi: The year 2019 was a turning point in the expansion of the project: I received funding from the CNRS’ Innovation program, and it allowed me to put together a research team and thus to increase the amount of data we could process, to refine my work on NLP algorithms for our databases, and to expand the project to fields other than brain science. We recently thought how to adapt our history program for World War II research. The task we are faced with in this case is the integration of very different kinds of data — testimonies, maps, displacements or travels, memories, data from military sites or camps. Some of the expertise we developed for this recent project was also used to evaluate the psychological and emotional state of the French population after the November 13, 2015 terror attacks in Paris. Our November 13 program aims to collect the testimonies of about 1,000 individuals who have a relatively strong social and geographical connection with the attacks. The task at hand in this case is to explore testimonies given in French, as interviews where individuals answer specific questions, but also as freeform testimonies. I am currently co-supervisor on a dissertation drawing on these testimonies to identify characteristics of post-traumatic stress disorder in textual documents. What is at stake here is whether a machine can identify or classify testimonies based on textual markers of post-traumatic stress disorder.
The challenges of interdisciplinary research
Salma Mesmoudi: It is important to report the problems we have run into within these interdisciplinary projects. For instance, the very last project I mentioned requires specialists in artificial intelligence — and specifically in deep learning for testimony analysis — but also specialists in linguistics to assist us in identifying textual characteristics. It also requires psychiatric expertise because we work on post-traumatic stress disorder, which is a pathology of the brain. That does not cover the social context of the event and its impact on individual and collective memory — both of which will also affect the testimonies we work with. This means that different fields will work together on the same project, but it is very difficult to ensure effective communications between such different fields. For example, terminology is problematic, because terms do not have the same definition in all these different fields. One of these words is “resilience”: whenever it is used in interdisciplinary seminars, it never fails to trigger intense discussions, since it is used differently in each field.
Another problem we have run into is the lack of funding for research based on open access data. Interdisciplinary research is often only possible if we use open access data (because acquiring new data is expensive). But we often have to justify why we would be using data which is available for free, and many researchers do not trust open access data. Yet, to me, this data is one of the great advantages of these projects. To progress towards European policies encouraging the use of open access data, we need projects like LinkRdata which show how open access data can contribute to research.
Making the best out of ChatGPT
Salma Mesmoudi: Everyone is talking about ChatGPT at the moment, and I think that we should use it rather than treat it as an enemy. I will thus try to identify how I could best use this technology for my project. In my opinion, it is crucial to educate people about new technologies. I will cite here a passage from Jean Piaget which is relevant and in fact remarkably fitting for our current predicament: “The principal goal of education is to create men [and women] who are capable of doing new things, not simply of repeating what past generations have done — men [and women] who are creative, inventive and discoverers, who can be critical, can verify, and not accept everything they are offered.” (Translation by Eleanor Duckworth.)
Our education system is good in some regards, but in others it should perhaps adapt to what science offers. What matters most to me in what Piaget said is the idea that we should not simply repeat what past generations have done. Of course, we have to know what they did in order to replicate it — but we must also go forward. So if we have tools that can assist us in synthesizing the past so that we can move forward, why not use them? The main advantage of ChatGPT could be pedagogical assistance. Let us look at an example: Google produces data. When we ask Google a question, or submit a keyword, it generates a list. It then falls on us to synthesize results from this list. But ChatGPT performs precisely this synthesis (by averaging) and gives us what is the most likely postulate. There are naturally inconvenients. When it does not find a suitable result, it makes it up! It thus integrates untrue elements. This is why it seems to me that its usage would be a significant help to develop critical thinking in the young generation.
Concerning artificial intelligence more generally, we are entering a new era, and we do not yet know what will change. We are thinking that some professions might disappear, and that others will be created. This is also a reason why I think that we should integrate AI in our education system, and support our students in this unfolding change. Let us consider the case of language courses. Have you ever used language learning applications based on artificial intelligence assistance? These applications will create personalized language courses. They follow the student’s own rhythm, introducing appropriate new material drawing on continuous tracking of their personal progress through live evaluations. Could we integrate this kind of applications — with the assistance and guidance of teachers — to improve student learning in general?
Meanwhile, artificial intelligence seems to create or force the creation of significant divides in several fields (like social science, cognitive science and economics). It thus becomes strikingly important to take our fears rationally — by observing these technological developments and tracking them. It seems essential to me that we have some form of international governance on algorithm development — and on data as well — to make sure that progress is shared. Artificial intelligence must be regulated, and more work and reflection about responsibility is required. For instance, in healthcare fields, we encounter problems with diagnostic responsibility. AI cannot be the one responsible for a diagnosis. The same is true for judgements in courts of law. We must also guarantee transparency for algorithms and data, so that we can know where each result and each development comes from, and what they are based on. To put it plainly, I encourage our institutions to have their own charters for AI use.