Interview with Marie Cottrell on Mathematics, AI and cross-disciplinary research
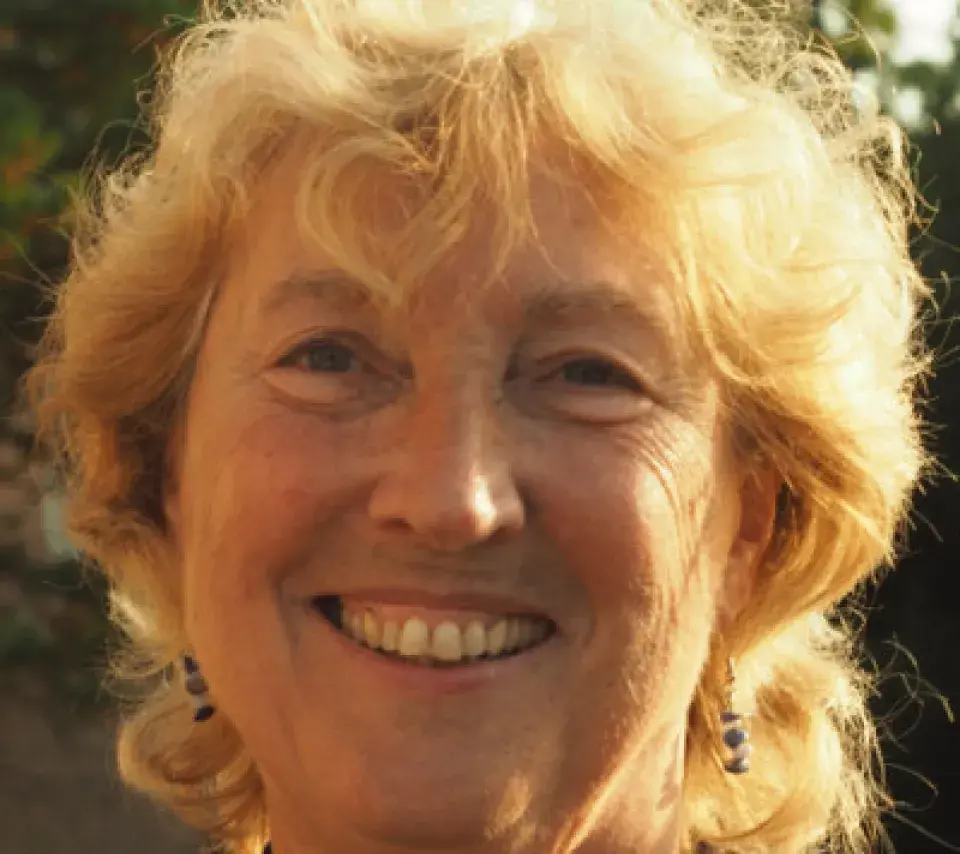
Marie Cottrell is Professor emeritus in mathematics and works in the fields of applied mathematics, statistics and neural networks. She is doctor honoris causa in mathematics at the University of Havana in Cuba and honorary doctor at Aalto University in Finland.
A former student of the École normale supérieure in Sèvres, she began her career as a high school teacher. She then held positions as assistant and master-assistant, then as an Associate Professor at the universities of Paris and Paris-Sud. She has been a Professor at Paris 1 Panthéon-Sorbonne University since 1989. Between 1970 and 1973, she was a Visiting Professor at the University of Havana. She was also the head of the SAMOS (Statistique appliquée et modélisation stochastique) research unit, ancestor of the current SAMM (Statistique, analyse, modélisation multidisciplinaire) research unit, from 1991 to 2012, and head of the TLDC second-year Master’s diploma from 1997 to 2010.
Could you tell us about your academic training and your interest for applied mathematics?
Marie Cottrell: I began with the training in mathematics which was typical in France in the 1960’s, that is, with so-called pure mathematics. At the time, probabilities and statistics were not taught in classes préparatoires, nor was numerical analysis. It was really only what we call pure mathematics today. When I graduated from the École normale supérieure, I taught in a high school (Lycée), and got a DEA (a Diplôme d’études approfondies, replaced today by the M2 second-year Master’s diploma) in logic. In 1967, I was hired as an assistant at the University of Paris, and I later obtained a transfer to the University of Paris-Sud. Then I left France to teach at the University of Havana in Cuba, where I found my colleagues already very much involved in work on applied mathematics. Computer science had yet to grow and develop, but it did exist — we were in the 1970’s. I understood how interesting it could be to use mathematics for all sorts of applications to real problems, to put them in the service of different studies and projects, etc. And this is how I developed an interest in applied mathematics and computer science (even though it was still far from being computer science as we have it in mind nowadays).
Returning from Cuba in 1973, I went back to teaching in a high school for a year. During that time, I changed course in my mathematical training and began taking classes in probability, statistiques and computer science in Jussieu. Studying in those fields was a true discovery for me. Indeed, in those days, French academic mathematics were still strongly influenced by the Bourbaki group, ie. focused on theory, axiomatization and on the development of theories which were to be as comprehensive as possible. After a year, I returned to Paris-Sud with a position as assistant, then as master-assistant. Then I started working directly in statistics: I got the DEA while doing supervised research in statistics, which greatly accelerated my training.
And what about Aalto University?
Marie Cottrell: I did not go to Aalto University right away — it comes later. There was a whole journey with Aalto. When I was in Orsay, at Paris-Sud, some researchers in our lab were intensely interested in all that was connected to the modelization of real biological systems, especially the visual system. It was a popular research topic between 1980 and 1985. Through this group, which studied the modelization of real neural systems in biology, with a special interest for the visual system, I took part in conferences, research collectives, etc. I also read papers on the self-organization algorithm defined by Teuvo Kohonen.
It was designed as an algorithm for the modelization of the sensory system — which is another real biological neural system. And so these researchers tried to “make equations” out of the way in which real neurological systems worked, starting from the idea that real neural systems are made of neurons joined together by synapses. There were plenty of questions to dig into: How could such systems work ? What was their equilibrium state ? Toward what did these real neural systems tend ? Specifically, could we modelize visual or sensory learning in the very first days of life ?
Thus we were in close contact with biologists, so that they could explain to us how real networks worked, as they could know it themselves with the instruments available at the time. Since then, these instruments have progressed so considerably that some things which they could not see, they can see now. But even at the time, we already had this idea that there were a large number of neurons connected together, and that should be modelizable in mathematical form using units joined together by connections — what we call neural networks today. And so this constant back-and-forth between real neurons and artificial neurons produced an enormous amount of studies and results, which made for an exciting atmosphere in the first half of the 1980’s. It was in these circumstances that I met and exchanged frequently with Professor Teuvo Kohonen, a Finnish researcher, working at what is now the Aalto University, and who unfortunately passed away in 2021. We did a tremendous amount of work on the properties of the Kohonen algorithm, always collectively, first in Orsay, and then more specifically with Jean-Claude Fort.
We gradually moved away from the modelization of real neurons and biological neural networks, and towards the modelization and mathematical study of an algorithm which itsef modelizes this process. At the time, Teuvo Kohonen was based in Espoo, a suburb of Helsinki, where Aalto University is now. We had frequent contacts with him, and his colleagues and students, both during scientific conferences and in correspondance. In 2012, Finnish colleagues offered me the title of honorary doctor of Aalto University.
Tell us about your early work on artificial intelligence in the 1980’s.
Marie Cottrell: Coming back from Cuba in 1973, I worked as a high school teacher again for a year; then I studied probabilities and statistics, teaching in both fields at the same time. In the 1980’s, two new teachers arrived in Orsay: Gabriel Ruget and Robert Azencott. They had an interest in modelization, and they led a research group and a seminar on issues relative to modelization. And so — around 1985, myself and other colleagues started to work on neural networks. At first, we focused on biological networks with the idea that we could mathematize them, ie. express them as equations in order to try and obtain rigorous results about their behavior. I also worked for five or six years in collaboration with Jean-Pierre Rospars, a biologist studying specifically the real network in the cerebellum. We co-authored a number of papers — and it was fascinating work because he would always try to get closer to biological reality, while we would always try to simplify. And he was fairly desperate because he thought we went much too far in terms of simplification. And then we tried to explain to him that we could not modelize every detail, molecule by molecule, like he was attempting to do. And that’s how we did some amount of work together between 1985 and 1990.
This is what led me to develop an interest in what we called multilayer perceptrons (MLPs), as well as in neural networks and Kohonen maps.
Precisely — could you tell us more about your work on artificial intelligence, Kohonen maps and neural networks?
Marie Cottrell: At the time, artificial intelligence was not the keystone in all of this. When we do a historical introduction to the topic for students, or in a seminar, we trace the work on artificial intelligence back to the middle of the 20th century, when the term was first used. Researchers then had a lot of questions about natural intelligence, and they tried to modelize it, to design a machine, an algorithm or some other thing which could mimick human intelligence. And so the term “artificial intelligence” existed as a complement or an opposite to human intelligence.
But AI was not much discussed in the 1980’s — it was a field which had reached somewhat of a limit. At the time, we were trying to express logical reasonings as rules and symbolic calculus, and we could quickly identify limits. So when I began to work with colleagues on artificial or biological neural networks, we did not really use this term — it was more of a historical term. (I’ll say that I find it very embarassing to say that “I” did all this, because I have always worked together with colleagues, be it in Orsay, at Paris 1 or in other places…) We were trying to make connections between what some of our colleagues were doing (in engineering, computer science, physics or biology) and mathematics. We tried to mathematize their results, that is, to give clear definitions of them, then to identify some connections between these notions and basic statistics. There was this language being developed by researchers working on neural networks, and we tried to “translate” it: for instance, we “translated” learning as estimation, and what neural network specialists called “synaptic weight”, we defined as parameters for the model. So yes — these connections between mathematics, statistics and the varied, multidisciplinary field of neural network research — yes, we worked quite a lot on that. What we did was to put a lot of effort into teaching and mathematizing this work. For example, we studied some theoretical properties of MLPs, wondering if these algorithms converged, ie. if they stabilized after a long period of time. Could these nonlinear algorithms be used as predictors, that is, could they be used in time series which are often studied based on linear models?
As for Kohonen maps — from a mathematical standpoint, they are the extension of a well-known algorithm: the k-means algorithm, or cluster centers algorithm. It dates back to the very beginning of the 20th century, or even to the late 19th century. On the one hand, Kohonen’s algorithm was developed in an environment having a lot to do with computer science. But on the other hand, statisticians reported that this algorithm was quite similar to something which had been known in their field for a long time. And we worked a lot to build bridges between fields. We were asked, for instance, to design courses for European projects in which we were mathematicians whose work it was to clarify notions and definitions. And we were especially tasked with having the statisticians understand what new ideas they could find in neural models, the same being true for the neural networks specialists. Kohonen’s algorithm itself is the area in which I did the most work (with Jean-Claude Fort and Patrick Létrémy at Paris 1, Michel Verleysen at Louvain; and Éric de Bodt at Lille). Kohonen himself was not a mathematician: he had these formidable intuitions, and he obtained very practical results — but he was always a little frustrated when he did not understand why his algorithm was working. So he was very happy that some mathematicians were trying to identify the theoretical properties in his algorithm. Conversely, when we showed that some of the properties he had identified were not quite accurate, he became vexed and angry. Now, since we had been working together for a long time, and were well acquainted, he was not that angry... There were persistent communication problems between our fields, which were not exactly similar. For instance, to him, if an algorithm converged nine times out of ten, then it was convergent. But, to us, the algorithm in this case cannot be said to converge: we cannot conclude that. Even if, when we come to practical scenarios, and we do the tests and transpose the algorithm into code, even if the algorithm performs well in those cases, we cannot really conclude that it is convergent. And we did have long and complex discussions about this—it was thrilling to see the connections between mathematics in the most rigorous sense (theorems, etc.) and some of the practical properties of these algorithms.
You have worked closely with humanities and social science fields. What did it contribute to your laboratory an your research?
Marie Cottrell: The collaborations I spoke of with biologists were active mostly while I held my position in Orsay. But when I came to Paris 1 as a professor, there were no biologists, no physicists, no engineers. Collaborations which were already on-going persisted, but it was harder to work together as effectively. To identify new research topics, I worked with a colleague, Patrick Gaubert, an economist. I also worked with Patrick Létrémy: we developed and studied algorithms which we used on practical cases before we applied them to economic data. This was like an extra toolbox, on top of the classical statistics which our colleagues used all the time, and had used for a long time. The main feature of these new tools was their being nonlinear models, which are more complex and can track the data better.
Since there were a lot of humanities and social science researchers at Paris 1, we worked with other fields, besides economics, like geography, specifically with Lena Sanders. It was very interesting, how our methods would interact. The very first project we did with her was about demographics in the Vallée du Rhône: we studied all the towns in the region, those which experienced demographical growth, those which experienced the reverse, etc. So that was a first project.
Then we began to exchange with historians. At first, these exchanges were pedagogical conversations with a colleague, Pierre Saly, who is retired now. Because there had always been a basic course in statistics for undergraduate history students. Pierre Saly had written a textbook — Descriptive Statistical Methods for Historians — drawing on his rich pedagogical experience. I read and re-read the textbook to discuss some things with him. This was an entry point for greater contacts with our other colleagues in history — especially with Stéphane Lamassé, who had an insatiable curiosity, a deep knowledge of statistical methods, and an interest in tools which were a bit more complicated, more modern and more recent.
This collaboration was very interesting because it was exciting to learn a little history while we were working on our models. It was the topic itself, its historical context which Stéphane would tell us about. It broadened the possible applications for my lab’s research. But this collaborative work also required a lot of time, since we needed to take the time to understand the problems we were dealing with. There were three or four of us working with the historians. We could not show up with our toolbox, and tell them: “These are the right tools, and that’s it.” First, we need to work through the context and interpret, and then the data itself is very important: it is not canonical. There is quantitative data and qualitative data, and there are gaps in the data. In historical research, data is not as impeccable as the INSEE (Institut national de la statistique et des études économiques) census for which we collect data each year, and for which we could even discard incomplete data because there is so much of it. For historians, when there is no data, there is simply no data: they cannot invent it.
I remember the work of Madalina Olteanu and Julian Alerini: there were a lot of gaps in their data, since they were accounting for administive documents published in the Savoie region. So there were months with no data, and then months with many documents all at once. This allowed Madalina to come up with a new method for data processing when there are lots of zeros. There are data items with numerical values, but it can also be punctuated with several data items with zero for a value. If we analyze and process this data like regular data, we will get very poor results. And so Madalina designed a method to analyze this data while taking the zeros into account. We also developed a method to simultaneously processs both quantitative and qualitative data, which are entirely different kinds of data. So we made the required theoretical advances in algorithm definition so that we could process both kinds of data. In classical statistics, what comes to mind first as data is data from the INSEE, or banking data, etc. — but data that we encounter in historical research is very different.
How has artificial intelligence evolved since the 1980’s — specifically concerning artificial neural networks and the specifics of neural methods?
Marie Cottrell: It bothers me to talk about artificial intelligence because, to me, the “artificial intelligence” that we hear about on the morning news — that is a misuse of words, a shortcut that we use to save time.
In our work on MLPs particularly (between 1980 and 2010), we were limited in a significant way by the speed of the algorithms developed at that time: they worked very well, but they were also very slow. When we were dealing with a lot of data, we were never sure whether we had reached balance or not. We never knew if we were done. With MLPs — what artificial neural networks were called then — researchers would sometimes start running a program, only to realize a week later that it was not fully stabilized yet, and then they did not know when to stop. Of course, a lot of work was done to share tips and ways to know when the program could be stopped. But it was extremely slow, and when we had a lot of data, it just jammed. Because at the time, and until about 2005 or 2010, computer storage and processing speed were not sufficient — despite efforts to work in parallel, to use storage in blocks, etc. And what really changed things, and led to deep learning and to artificial intelligence skyrocketing, was the development of computation speed and storage capacity. Meanwhile, in the actual world, the amount of data which we could have to process became massive — it was multiplied thousands, perhaps millions of times even. And so I think that this is what changed most fundamentally, because, in fact, the ideas, and the aims, and all the tasks which people could want to perform did not really change. It’s simply the scale — this has changed wildly. Given this change in scale, some things look much more accessible now than they did before. And since the basic problems were mostly worked out, a lot of new theoretical questions surfaced which are studied intensely now.
For instance, there is what we call “sparsity”. Before when you had 25 variables, you kept all of them. You were telling yourself that you would keep all the information you had, except if you had something which was truly and evidently useless. Take INSEE data, for example: we had this habit of removing some number of variables, since we often had the number of men, the number of women and the total number of people. We figured that it was not necessary to keep all these numbers, and we removed one of the three variables, as it was plainly a function of the other two. But save for cases like this, we tried to keep all the information we had. Now, from the moment when we have data with thousands of descriptors, it makes no sense to keep all of it. Interpretation will be much too hard. We will not be able to describe what we obtain properly. So we try to make sparse models, that is, to remove the descriptors which we think are not that significant for data description. And so researchers develop algorithms which try to remove variables — but not randomly: only those variables which are not very meaningful. This has led to important work.
Another distinction to mention — before, both in classical statistics and in work on neural networks, we assumed that the data observed was stable in time. We assumed the underlying model for it remained the same. Even if we noticed some temporal evolution, we modelized it with equations which were valid throughout. But in reality, there are ruptures. And so research on these breaking points is also very important. The simplest case is the one in which you have only one time-dependent variable, for which you try to determine where change — ie. a rupture — took place. What is a most complicated case — and this is a fertile area of research at the moment — is having 100 variables at the same time, and searching for breaks in the whole set of 100 variables. A colleague of mine, Alain Célisse, at the SAMM, works on these multidimensional breaks with Madalina Olteanu as a collaborator — who was an Associate Professor at Paris 1, and is a Professor at Dauphine since last year.
It is hard for me to tell you what really changed. There were technological advances: we built different computers, servers and processors… And since researchers had solved some basic problems, they turned to more complex problems which they could not tackle before, but which they can try to solve given the new, more massive computation capacities.
But we are not confined to fundamental research — we also work on applied science. Based on these methods, algorithms and how-know, SAMM has been collaborating with the enterprise Safran since 2008, working with engineers who study the realiability of airplane engines — which is fairly useful in day-to-day life! It is a service Safran provides to help experts identify engine failures or outages, and thus prevent them entirely before they happen. This is what we call “health monitoring”: we try to assess how healthy an engine is. We regularly work with Safran engineers through postdoctoral positions or CIFRE grants (Convention industrielle de formation pour la recherche). In fact, the know-how concerning what we can call artificial intelligence — but which we call algorithmic methods — is developed, applied and perfected by working on engine monitoring data, obtained either during simulation, or during actual flights. And so, to us, artificial intelligence is not mechanical at all — it is not a black box. On the contrary, we develop algorithmic methods, we test them in concrete situations, we run simulations, we work on different properties, and then, as much as we can, we try to prove theoretical results based on these methods. Specifically, we will try to know: Does the algorithm converge? What is the quality of the estimators provided? How can we compare these methods with existing methods? Are they an improvement compared to classical statistical methods?
You are also a text and data mining expert. Could you tell us more about this field and the issues surrounding it?
Marie Cottrell: To be honest, I am no text mining expert per se. But during our collaboration with Stéphane Lamassé — who possesses vast knowledge about text mining based on “mostly traditional” statistical methods — we encountered problems of text classification. Specifically, we worked on medieval mathematics textbooks, and we tried to group them, to situate them in relation to each other, etc. In general, with historians and in humanities and social science research, we have data with which we are not used to work. Although text mining has always been an important field for historians, it involved traditional methods, which served to calculate word frequency, then to create contingency tables for various words and texts to identify the frequency at which this or that word occurred in this or that text. We tried to use Kohonen maps, that is, a classification method maintaining the neighbouring structure in observations. And so we obtained results which made our colleagues in history quite happy. They allowed them to see interpretations they had not initially seen, and to formalize intuitions which they had but could not justify. We managed to generate maps (of a sort) in which “similar” texts were represented as neighbours, while texts which were at two ends of the map were very different texts. I do recall we had differentiated texts which were purely academic from texts whose purpose was more practical, like textbooks for shopkeepers to teach them calculations and accounting. Our colleagues in history were pleased — and so were we, even though the interpretation of those results belonged to them, and only they could tell us if what we had obtained meant anything and could work as a support for commentary. I did not work a lot in text mining. What I did was essentially through this collaboration with Stéphane Lamassé and his team.
France recently launched a strategic endeavor in which AI plays a central role. According to you, how can a university with a strong social science focus such as Paris 1 Panthéon-Sorbonne be a part of this project?
Marie Cottrell: I think that we must be extremely careful and rigorous here, as it is easy to throw words around: it is not because we say that France launched a great project about algorithms that the current situation will progress. I think that what we can do is to be as humble as we can, and teach our students how to be as humble as they can, and as rigorous too. Because saying that we have an AI orientation can mean absolutely anything. For a very long time, statistics were criticized on the ground that we could have them say anything and everything — well, that is even truer with artificial intelligence. We need to do this exactly like we do it in statistics courses: show our students that we cannot take conclusions to be true before they have been proven, and remind them to proceed with caution and rigor. It is the same for so-called artificial intelligence: we have to be as precise as possible. Now — I speak as a mathematician, but of course artificial intelligence is also of interest to philosophers, who will have a philosophical perspective on these matters. As a mathematician and a statistician, one has to be extremely humble, explain the basics and teach them, give the meaning of key terms and connect them with the right notions. We have to explain to our students that an algorithm never does anything other than what you asked it to do. So we will not discover anything magical by using one algorithm rather than another. Algorithms, if they are well-coded — we will ask it to do something and it will do it. And indeed, it will be able to perform calculations which humans cannot do and do them much faster; it will identify structures, groups, relations and graphs in databases so phenomenally large that humans would drown in them. That is true — but really, all algorithms do is to perform tasks for which they have been programmed. It is truly dangerous to think that artificial intelligence has a life of its own, that it will help everyone do anything for better or for worse. That is a fantasy through and through. What we have to do is help our students know what they are talking about, and know it well, help them be humble, bring them back to basics, and finally help them not to take results which are sometimes impressive to be magic. Purely and simply, algorithms process — they summarize data, huge amounts of data (something which humans would find very difficult to do concretely, if they could do it at all). Algorithms will discover structures invisible to the naked eye, but they discover those structures only because you asked them to look for them. There is no magic in artificial intelligence. And this, I think it is crucial for students to know with conviction — and not only students, but the general public as well.
Since at least the 1990’s, we have trained Paris 1 students in artificial intelligence without giving them any course with that name, since their courses are about neural networks and algorithms. We show them how various mathematical techniques can perform various tasks (like classification, prediction, data simplification, or graph construction). And these are all tools which we now add on top of older tools, which were also data mining tools, even if we did not use this word back then. For example, when you calculate an average, you are doing data mining. You are doing data simplification, you are doing data mining — but, of course, it is not very good. Statisticians have designed a lot of methods to extract information from large databases. And so data mining continues on this path, and we are adapting our tools and techniques for millions of data items, since we cannot use methods that are too simple. We have to use other methods. Iterative methods are specifically interesting. When we studied statistics in high school, we were often doing exact calculations — we took all the data in one swoop, and we made one calculation. Nowadays — with all that we call machine learning, and data mining — we know to take data packets, and sometimes we consider each data point separately, and we make calculations that come close to a result: there are iterative calculations. This notion of iteration is very closely connected with the notion of algorithm. We need to make sure our students understand it well, so they can also understand that it is not always best to try and have all the data, and do exact calculations. Because we will not make it. There are some cases in which an exact calculation is not possible, and so we make successive approximations. And that is also very important to teach.
Another notion which we have to insist on with our students is simulation, that is, before we start studying real data and before we can know whether our algorithm works well, it is often quite useful to start by running simulations to experiment. It is a lot like experiments we would run in a lab, but they are numerical experiments aiming to understand how to adjust our parameters for the whole thing to work. And so it is a way of doing mathematics which can be difficult to grasp for our students — who sometimes see mathematics as an activity where we take a piece of paper and a pen, and we do some calculations and — there — we have a result.
What would be great would be for all our students (in law, languages, humanities, social science, etc.) to have a bit of a different idea of what mathematics are about, so that they stop thinking that mathematics are a fossilized field in which everything has been proven already. A lot of non-mathematicians among our students think that nothing is left to prove in mathematics, that it has all been done, that it is a closed, fixed science. They struggle to understand (or do not understand) what is research in mathematics, and so how it happens that mathematics interact with other fields. It is something we very much want them to acquire in university. For instance, if I consider the case of historians, I recall heated discussions I had with colleagues in history who were telling me that we should not introduce quantities in history, that statistics truly were the opposite of historical science. And I was there, trying to tell them that it all depends on the way we use them, and that indeed there had perhaps been authors who had used statistics to support anything and everything. Likewise, we can use artificial intelligence to support anything we want to say. But this is not a feature of mathematics; it is improper use of data and material. And so it would be good if our students had the scientific culture required to think critically about these issues. It is the same thing concerning ChatGPT: it works well to fix spelling mistakes, or to find a specific answer to a specific question you might have. It works well to write computer code when we already have some pseudo-code, and an idea of the steps involved. It will indeed put it in the proper format. But it is not magic, and ChatGPT will not discover anything which is not already in the human mind. What it is is simply a tool which will allow us to go faster, and to synthesize. It is fueled only by the content humans have given it and still give it as input. I think it is very important for our students to be able to think critically about this. I am not saying that they should all be taking special coursework on the topic — but rather that they should at least be critical and be able to situate these ideas which are frequently discussed in various media.
The great speeches and the various promises we currently hear about artificial intelligence do frighten me a little, because I think there is a risk of creating illusory expectations, and also some risk to feel obligated to obey some commands based on the fact that artificial intelligence formulated them. But that is very dangerous, because artificial intelligence does not formulate or say anything. If you use artificial intelligence tools properly, and if you ask questions, well then, you will get answers to your questions, and that is all. It is exactly the same as the recommendation systems used in marketing and quite well-known. This system is a human invention, and it absolutely does not predict what you will like or will not like. There is no reason why we should follow these recommendations as if they were divine commandments, or possessed superior authority. The risk is for AI to be used by some to profess injunctions, or to pretend that they know some superior truth, while all AI should be is a tool at the service of human society, to help us with complex or tedious tasks.
It is good that the Artificial Intelligence Observatory was set up at Paris 1, because it will allow colleagues in philosophy, economics, history, computer science and mathematics (who have all worked separately on AI) to meet and talk. Artificial intelligence is good if we use it critically or collaboratively, but it is not magic.